Bayesian deep learning, overview and challenges
Julyan Arbel
April 16, 2025 11:00 am
INRIA Grenoble
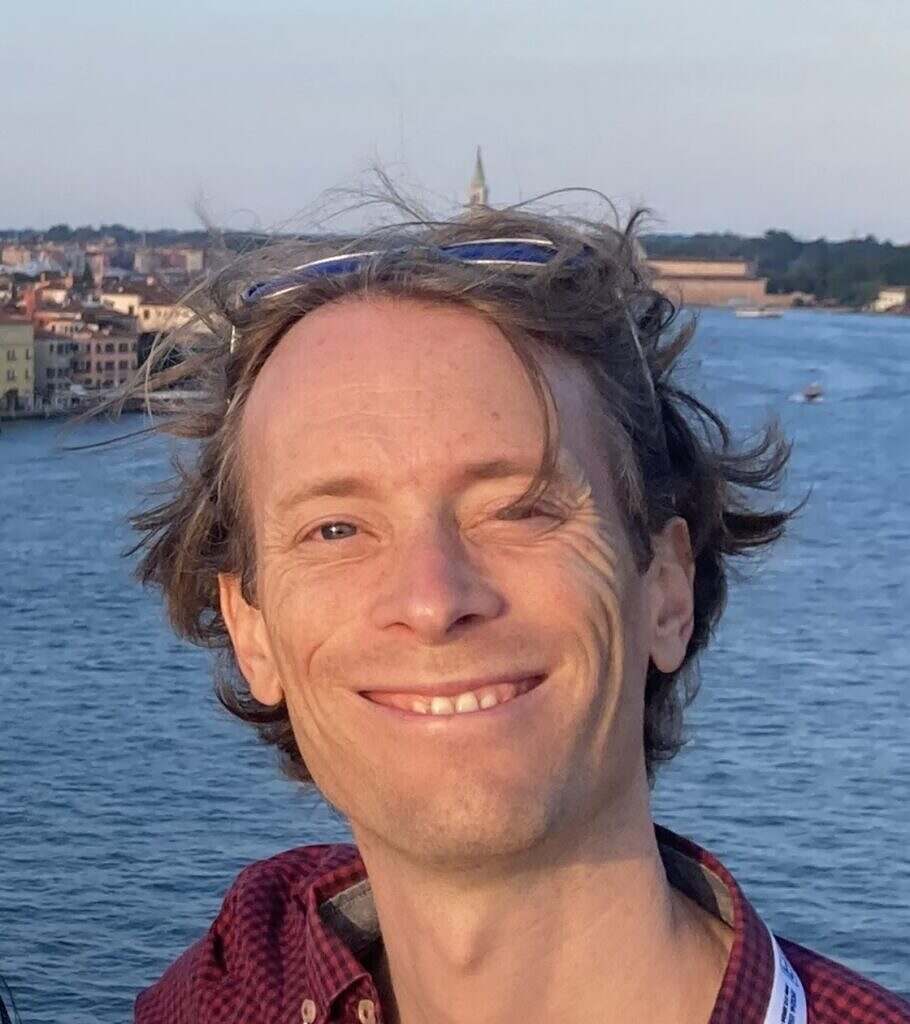
Julyan Arbel is a researcher at Inria Grenoble with expertise in Bayesian deep learning and Bayesian nonparametrics. He holds a Ph.D. in Statistics from Université Paris-Dauphine and an HDR from Université Grenoble Alpes. He completed a postdoctoral fellowship at Bocconi University in Italy and was later a visiting researcher at the University of Texas at Austin.Beyond his core work in Bayesian deep learning and nonparametrics, his research spans a range of topics, including the theory of sub-Gaussian and sub-Weibull distributions, Approximate Bayesian Computation, variational inference, MCMC, bandits and sequential learning, as well as extreme value theory and risk measures, with applications in ecology, bioinformatics, and neuroimaging. His publications appear in leading journals and conferences across statistics and machine learning.He has contributed to the community through the organization of workshops and conferences, and by serving on editorial boards and program committees. As a supervisor, he has mentored over 20 PhD students and postdoctoral researchers who have pursued careers in both academia and industry.
Bayesian deep learning is appealing as it combines the coherence and natural uncertainty quantification of the Bayesian paradigm together with the expressivity and compositional flexibility of deep neural networks. It has its roots in pioneering work from the 1990s on Bayesian neural networks. Its strengths lie in principled uncertainty quantification, improved data efficiency, and adaptability, making it impactful in safety-critical fields like healthcare and autonomous systems. In this talk I will provide an overview of Bayesian deep learning and discuss some of the key challenges the field faces in addressing modern machine learning problems.
Archive