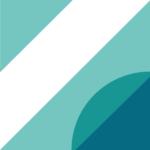
Language
How did language evolve? This question is among the great unsolved mysteries and a fundamental scientific challenge. We investigate ways in which artificial agents can self-organize languages with natural-language like properties and how meaning can co-evolve with language. Our research is based on the hypothesis that language is a complex adaptive system that emerges through adaptive interactions between agents and continues to evolve in order to remain adapted to the needs and capabilities of the agents. We explore this hypothesis by implementing the full cycle of speaker and hearer as they play situated language games and observing the characteristics of the languages that emerge.
How did language evolve? This question is among the great unsolved mysteries. Other big questions about our nature as human beings and our place in the universe have at least tentative approaches to answers. Questions like where the universe came from, how life evolved, how human beings evolved as a species… most people can frame at least a rough response. They have a grip on these questions. The question of the origin of language – that capacity perhaps most characteristic of our species – remains mysterious. This is a fundamental scientific challenge.
At the Sony Computer Science Laboratories a project has emerged over the last decade which tackles this question directly and offers the possibility of an answer. We explore the evolution of human language through the interactions of communities of autonomous robots over many cycles of interaction. The robots have capacities which we put in – both hardware and software. They have perceptual capacities, for example they may be able to hear and distinguish sounds, detect gestures. They can move, interact. And we can put in different software determining for example how they react to items they don’t recognize, how they respond to deviation from a pattern, and much more. We choose sets of parameters and set communities running over cycles of many thousands of interactions. From these simple interactions, and the individual responses of agents, complex systems arise which actually have the features of communication in natural languages.
This project involves achieving vast goals, including the core goal of formalizing natural language, to be able to model it. The challenge here is the sheer complexity of the whole of what makes up language, from the acts of hearing, producing sound, articulation and interpretation, parsing, incompleteness, error, the speed at which the whole process takes place. A normal adult speaker probably knows at least 100 000 units of language construction, which are manipulated in varying conditions of audibility, success and failure, with language speakers from a similar dialect group or very different, very rapidly. Yet we manage to communicate. Moreover, we innovate in communication. The complexity and multiple parameters influencing transmission of meaning are not the only barrier to creating a successful model. Language changes, shifts, modifies, is dynamic. New meanings arise, are shared, catch on, spread, die out – sometimes very rapidly, or sometimes, as in profound changes in a language structure, over thousands of years. The dynamism, the fluidity of language, must be captured in a successful model. How is it possible that agents can innovate, introduce new meanings, and these meanings can come to be shared? How is innovation possible?
After more than a decade of research CSL teams working together with the VUB AI laboratory have built a formalism (called FCG) that works, that encompasses these features, and moreover that is available to other researchers. Features described below suggest the richness of the data that can now be mined using this methodology and the perspectives for research that open up.
Why are robots the right solution for embodying the experiment? Why is modeling the problem with robots interesting?
The agents are able to act, interact and perceive – the basic situation of human beings prior to communication through language. Moreover, all these aspects of their capacities can be modified by the experimenter, for example how they process sound and “hear”. This gives the opportunity for testing different hypotheses about what might be necessary – what we might have to have in place – for language to be possible. Obviously, those capacities have to be embodied in the robots, which is a whole separate programming challenge – to have agents which are capable of perceiving the world, or responding to the world. A second key achievement of Sony CSL has been development of a framework called IRL link which relates the agents to the world through their sensory-motor apparatus.
Why robots not people?
Apart from the fact that they can be set up with chosen parameters, and start with a “clean slate”, there are two game-changing advantages: number, and time. The communities of agents can be set up with hundreds of agents, to interact over thousands of cycles of interaction, as the experimenters call it, “cooking” the experiment over a long period of time. This gives us access to peering into the dynamics of change which represent in human terms hundreds of thousands of years. We can truly turn the clock back. To give an idea of the slowness of change of some of the strata of language: the shift from the Old German case system to Modern German is an event that started around the year 1100 and is still continuing (link). With this methodology we can run simulations to try and understand the forces at work that are bringing about this change. And crucially, these experiments are scientifically rigorous. Any other researcher can repeat them. In short, using this methodology the experimenter has powerful control over the capacities of the agents, plus the ability to make large numbers of them interact over very large time cycles, producing results that can be replicated by other experimenters. The excitement surrounding this project is the possibility of using this new methodology to actually make concrete progress in understanding the evolution of language. Several insights and resources are key to the success of this methodology. The major breakthrough is the vision of the potential combined power of these tools when applied to the question of the origin of language. They include the following:
Thoughts about simplicity and complexity
A key insight here is that advances in understanding complex systems from other disciplines – in particular evolutionary biology – can shed light on the origins of language. Consider our recent understanding of how communities of ants, for example, can create nests, or chains to transport food. There is no central organization, no prior planning. Each individual ant makes simple iterative steps involving touching, pheromones, movement. The goal is achieved through self-organization from the bottom up, not imposition from above. Local alignment of individuals results in global coherence, in a giant act of collective bootstrapping.
A key aspect of this insight is also that from simple interactions agents can come to have shared understandings. They can learn, can acquire new concepts from other agents. This is fundamental to what language actually is: a system of shared meanings which make it possible to communicate.
Moreover, modeling the evolution of language in this way gives us the tools to model the dynamism of natural languages. Variation, innovation, emergence of new structures, their adoption, spread and possible elimination can be understood within this model (link to Experiments in Cultural Language Evolution). Which holds the promise that invention of language itself can be modeled.
Experimental situation
We have the possibility of putting this insight to work. We have the key elements of hardware, software and community. We have robots with wide-ranging perception capacities, and capacities for generating sound, gesture, reacting. Over a decade of work we have produced softwares for modeling language, and for enabling agents to perceive. With a multiplicity of agents we can simulate a community interacting over time. We have a community of autonomous, self-organizing agents whose interactions over time produce features and structures of natural languages.
Computational power
We now have the tools to model. Hence the relevance and power of this methodology. What we are talking about is so immensely complex that only now do we have the computational power to make models and run simulations that can mimic natural language. Moreover, since we can model, our results are rigorous. They can be tested by other experimenters, are scientific.
Richness of data
This approach has an immense richness of material to draw on, ranging from historical linguistics to anthropology to behavioral science. There are enormous amounts of rich data to build on, which have been extensively studied, and which can be fed into these simulations. The collapse of case structures from Old German to Modern German, the appearance of articles in European languages 2 000 years ago, the differential spectra of color recognition between different languages. We are just beginning to probe the potential for this methodology.
And the results are also very rich – they feed into one another. Each part adds more than just that piece of knowledge. We can come to understand how articles come into a language or how other structures collapsed – but the understanding we gain is much wider than that. Each experiment adds to the whole body of knowledge, and can be re-used in other experiments The research is cumulative – tools developed can be used in other experiments.
The combining of insights and technology at this time holds the promise of understanding the forces at play underlying language. The very mechanisms of our humanity.
Obviously, the research carried out has far-reaching practical/commercial applications, ranging from building interfaces that are meaning-driven, new techniques for accessing information or finding meanings in data, or human learning and tutoring, to name but a few.
But there is an excitement itself in asking the question – as physics was able to articulate a theory that took us back to the origins of the Universe – Can we turn back the clock on language? Can we return, in these experiments, to the roots of language, the time before language existed, and find out what had to be in place for language to evolve.
The future research of Sony CSL in the area of language is building on the results achieved and exploring this deep question for our understanding of ourselves.
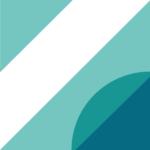
Language
How did language evolve? This question is among the great unsolved mysteries and a fundamental scientific challenge. We investigate ways in which artificial agents can self-organize languages with natural-language like properties and how meaning can co-evolve with language. Our research is based on the hypothesis that language is a complex adaptive system that emerges through adaptive interactions between agents and continues to evolve in order to remain adapted to the needs and capabilities of the agents. We explore this hypothesis by implementing the full cycle of speaker and hearer as they play situated language games and observing the characteristics of the languages that emerge.
How did language evolve? This question is among the great unsolved mysteries. Other big questions about our nature as human beings and our place in the universe have at least tentative approaches to answers. Questions like where the universe came from, how life evolved, how human beings evolved as a species… most people can frame at least a rough response. They have a grip on these questions. The question of the origin of language – that capacity perhaps most characteristic of our species – remains mysterious. This is a fundamental scientific challenge.
At the Sony Computer Science Laboratories a project has emerged over the last decade which tackles this question directly and offers the possibility of an answer. We explore the evolution of human language through the interactions of communities of autonomous robots over many cycles of interaction. The robots have capacities which we put in – both hardware and software. They have perceptual capacities, for example they may be able to hear and distinguish sounds, detect gestures. They can move, interact. And we can put in different software determining for example how they react to items they don’t recognize, how they respond to deviation from a pattern, and much more. We choose sets of parameters and set communities running over cycles of many thousands of interactions. From these simple interactions, and the individual responses of agents, complex systems arise which actually have the features of communication in natural languages.
This project involves achieving vast goals, including the core goal of formalizing natural language, to be able to model it. The challenge here is the sheer complexity of the whole of what makes up language, from the acts of hearing, producing sound, articulation and interpretation, parsing, incompleteness, error, the speed at which the whole process takes place. A normal adult speaker probably knows at least 100 000 units of language construction, which are manipulated in varying conditions of audibility, success and failure, with language speakers from a similar dialect group or very different, very rapidly. Yet we manage to communicate. Moreover, we innovate in communication. The complexity and multiple parameters influencing transmission of meaning are not the only barrier to creating a successful model. Language changes, shifts, modifies, is dynamic. New meanings arise, are shared, catch on, spread, die out – sometimes very rapidly, or sometimes, as in profound changes in a language structure, over thousands of years. The dynamism, the fluidity of language, must be captured in a successful model. How is it possible that agents can innovate, introduce new meanings, and these meanings can come to be shared? How is innovation possible?
After more than a decade of research CSL teams working together with the VUB AI laboratory have built a formalism (called FCG) that works, that encompasses these features, and moreover that is available to other researchers. Features described below suggest the richness of the data that can now be mined using this methodology and the perspectives for research that open up.
Why are robots the right solution for embodying the experiment? Why is modeling the problem with robots interesting?
The agents are able to act, interact and perceive – the basic situation of human beings prior to communication through language. Moreover, all these aspects of their capacities can be modified by the experimenter, for example how they process sound and “hear”. This gives the opportunity for testing different hypotheses about what might be necessary – what we might have to have in place – for language to be possible. Obviously, those capacities have to be embodied in the robots, which is a whole separate programming challenge – to have agents which are capable of perceiving the world, or responding to the world. A second key achievement of Sony CSL has been development of a framework called IRL link which relates the agents to the world through their sensory-motor apparatus.
Why robots not people?
Apart from the fact that they can be set up with chosen parameters, and start with a “clean slate”, there are two game-changing advantages: number, and time. The communities of agents can be set up with hundreds of agents, to interact over thousands of cycles of interaction, as the experimenters call it, “cooking” the experiment over a long period of time. This gives us access to peering into the dynamics of change which represent in human terms hundreds of thousands of years. We can truly turn the clock back. To give an idea of the slowness of change of some of the strata of language: the shift from the Old German case system to Modern German is an event that started around the year 1100 and is still continuing (link). With this methodology we can run simulations to try and understand the forces at work that are bringing about this change. And crucially, these experiments are scientifically rigorous. Any other researcher can repeat them. In short, using this methodology the experimenter has powerful control over the capacities of the agents, plus the ability to make large numbers of them interact over very large time cycles, producing results that can be replicated by other experimenters.
The excitement surrounding this project is the possibility of using this new methodology to actually make concrete progress in understanding the evolution of language.
Several insights and resources are key to the success of this methodology. The major breakthrough is the vision of the potential combined power of these tools when applied to the question of the origin of language. They include:
Thoughts about simplicity and complexity.
A key insight here is that advances in understanding complex systems from other disciplines – in particular evolutionary biology – can shed light on the origins of language. Consider our recent understanding of how communities of ants, for example, can create nests, or chains to transport food. There is no central organization, no prior planning. Each individual ant makes simple iterative steps involving touching, pheromones, movement. The goal is achieved through self-organization from the bottom up, not imposition from above. Local alignment of individuals results in global coherence, in a giant act of collective bootstrapping.
A key aspect of this insight is also that from simple interactions agents can come to have shared understandings. They can learn, can acquire new concepts from other agents. This is fundamental to what language actually is: a system of shared meanings which make it possible to communicate.
Moreover, modeling the evolution of language in this way gives us the tools to model the dynamism of natural languages. Variation, innovation, emergence of new structures, their adoption, spread and possible elimination can be understood within this model (link to Experiments in Cultural Language Evolution). Which holds the promise that invention of language itself can be modeled.
Experimental situation
We have the possibility of putting this insight to work. We have the key elements of hardware, software and community. We have robots with wide-ranging perception capacities, and capacities for generating sound, gesture, reacting.
Over a decade of work we have produced softwares for modeling language, and for enabling agents to perceive.
With a multiplicity of agents we can simulate a community interacting over time.
We have a community of autonomous, self-organizing agents whose interactions over time produce features and structures of natural languages.
Computational power
We now have the tools to model. Hence the relevance and power of this methodology. What we are talking about is so immensely complex that only now do we have the computational power to make models and run simulations that can mimic natural language.
Moreover, since we can model, our results are rigorous. They can be tested by other experimenters, are scientific.
Richness of data
This approach has an immense richness of material to draw on, ranging from historical linguistics to anthropology to behavioral science. There are enormous amounts of rich data to build on, which have been extensively studied, and which can be fed into these simulations. The collapse of case structures from Old German to Modern German, the appearance of articles in European languages 2 000 years ago, the differential spectra of color recognition between different languages. We are just beginning to probe the potential for this methodology.
And the results are also very rich – they feed into one another. Each part adds more than just that piece of knowledge. We can come to understand how articles come into a language or how other structures collapsed – but the understanding we gain is much wider than that. Each experiment adds to the whole body of knowledge, and can be re-used in other experiments The research is cumulative – tools developed can be used in other experiments.
The combining of insights and technology at this time holds the promise of understanding the forces at play underlying language. The very mechanisms of our humanity.
Obviously: the research carried out has far-reaching practical/commercial applications, ranging from building interfaces that are meaning-driven, new techniques for accessing information or finding meanings in data, or human learning and tutoring, to name but a few.
But there is an excitement itself in asking the question – as physics was able to articulate a theory that took us back to the origins of the Universe – Can we turn back the clock on language? Can we return, in these experiments, to the roots of language, the time before language existed, and find out what had to be in place for language to evolve.
The future research of Sony CSL in the area of language is building on the results achieved and exploring this deep question for our understanding of ourselves.
- Language
- Language
- Language
- Language
- Infosphere
- –Language
- Language
- Language
- Language
- Language
- Language
- Language
- Language
- Infosphere
- –Language
- Language
- Language
- Language
No results found.
- Language
- Language
- Language
- Language
- Language
- Infosphere
- –Language
- –Music
- –Sustainability
- Infosphere
- –Language
- –Music
- –Sustainability
- Infosphere
- –Language
- Infosphere
- –Language
- Language
- Infosphere
- –Language
- Infosphere
- –Language
- Language
- Language
- Language
- Language
- Language
- Infosphere
- –Language
- –Music
- –Sustainability
- Infosphere
- –Language
- –Music
- –Sustainability
- Infosphere
- –Language
- Infosphere
- –Language
- Language